Automating document review with 98% accuracy
We used Natural Language Processing (NLP) to automate internal review of RFPs, RFIs and security questionnaires, reducing time and costs associated with the process.

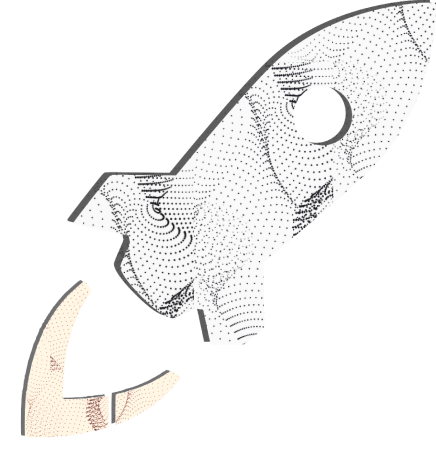
The Client
A company developing software which streamlines and simplifies the process of responding to requests for proposals, requests for information, and security questionnaires. Targeted to enterprises, the platform aggregates content in a centralized library, making it accessible and enabling collaboration across multifunctional teams.
The Challenge
The client’s platform offers a language processing functionality aimed at intelligently identifying and reducing content duplication, enabling document structure extraction and generating structured content from a free-form text.
Due to document formatting, file format and document context, enabling desired functionality requires constant human intervention, making the process costly and error-prone.
The Solution
Crater Labs developed a natural language processing (NLP) model for classification and segmentation of unstructured text of highly variable length. The model achieved 98.2% accuracy and greatly reduced the need for human intervention.
IP Generated
Crater Labs created a novel fully-convolutional network (FCN) enabling a larger receptive field for context extraction, with an iterative feeding mechanism in order to support better predictions through more stable gradient updates. The approach resulted in an exponential increase in the size of the convolution window as a function of network’s depth while maintaining stable gradient updates, which, in turn, enabled improved context extraction within the confines of a causal network.
Benefits & ROI
The solution enables the client to generate significant cost savings from automating a semi-manual process and reducing the need for human interaction. The resulting IP positively impacted the company’s valuation at their latest round of funding, resulting in lesser dilution of ownership for the company’s founders and original investors. In addition, the project generated double-digit return on investment from leveraging Canadian investment tax credits.
Subscribe
Sign up with your email address to receive news and updates
More Moonshots Worth Celebrating
Optimizing product placements in print flyers to maximize revenue
We developed a custom region convolutional neural network that provides key insights on how to maximize revenue with flyer layout, with a double-digit return expected within the first two years.
Read More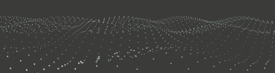
Saving $80K+ with employee questionnaire automation
We built an NLP-based neural network designed to automate question generation, saving $80K+ in the first year for one of Canada’s leading HR software businesses.
Read More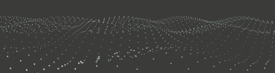
Automating document review with 98% accuracy
We used Natural Language Processing (NLP) to automate internal review of RFPs, RFIs and security questionnaires, reducing time and costs associated with the process.
Read More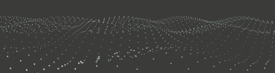